Generative AI, with its ability to create content, designs, and much more, has had quite an impact. It’s important to step back and assess its tangible impacts in the business world. High expectations surround this technology, yet actual implementations that deliver value are not as widespread as one might anticipate. This disconnect urges a closer look at how businesses can effectively harness Generative AI’s capabilities.
Bringing Generative AI from the lab to the market involves understanding the subtleties of its practical applications. Companies are coming to terms with the need for a robust data strategy that can seamlessly incorporate AI into existing processes. This demands not only technological readiness but also a cultural shift towards innovation.
There are shining examples of Generative AI adoption that serve as beacons of what is possible. In sectors such as pharmaceuticals, to personalized content creation in marketing, Generative AI is demonstrating quantifiable benefits. Realistic use cases like these showcase how weaving AI into the fabric of business operations can be a game-changer.
Looking forward, the integration of Generative AI into data strategies and operational frameworks is expected to expand. Crucially, businesses must remain keenly aware of the evolving landscape, stay adaptable, and acknowledge that a leap from potential to performance requires more than just technological prowess.
Streamlining Success: The Industrialization of Data Science
In recent years, the data science field has witnessed a significant shift. No longer are data models the sole province of highly specialized experts working in silos. Today, there’s a decisive move towards the industrialization of data science, which mirrors the assembly line innovation that revolutionized manufacturing a century ago.
What does this mean? Simply put, the production of data models is becoming more systematized. Organizations are now focusing on creating repeatable, scalable, and sustainable processes. This evolution is largely driven by advancements in Machine Learning Operations (MLOps) systems and the deployment of automated tools designed to streamline the creation and maintenance of data models.
These changes are about boosting efficiency and making data science more accessible. By investing in platforms that support the industrial model, businesses are able to ramp up productivity, thereby allowing data science to permeate various aspects of their operations. It’s a game changer that democratizes the value of data science across industries, from healthcare to finance to marketing.
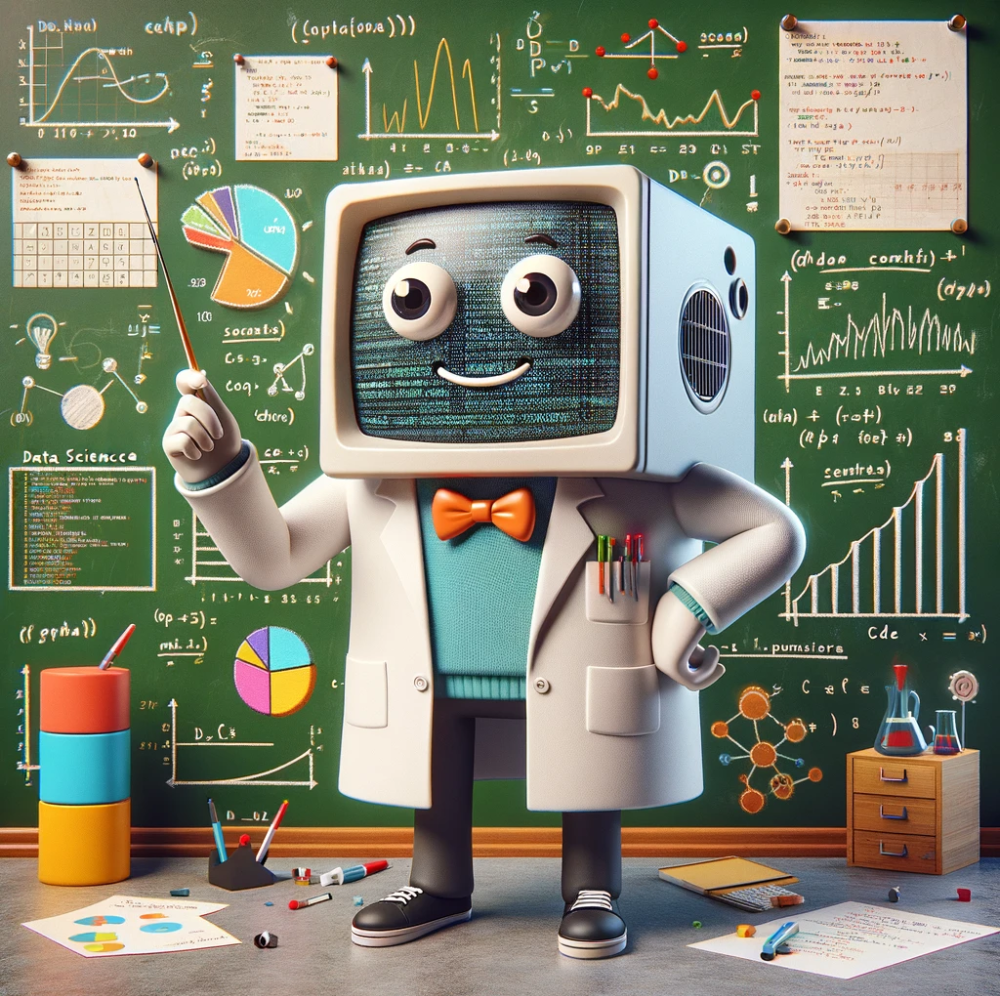
Take, for instance, a global retail company that implemented a platform approach to data science. By doing so, they managed to shorten their model development cycle, quickly adapting to market trends and customer behaviors. This isn’t an isolated incident. A growing number of organizations are reporting similar gains, proving that the push towards industrialization isn’t just a theoretical exercise—it’s yielding tangible benefits.
But this transformation comes with its own set of challenges. As the making of data models becomes more industrialized, the roles and skills demanded by the industry are shifting. The next section will discuss the rise of data products and the evolving roles within the field of data science, shedding light on the implications for practitioners and businesses alike.
The Evolution of Data Management and Roles
In the landscape of data science, the rise of data products represents a significant shift. These bundled offerings of data, analytics, and AI serve as powerful tools that organizations leverage for decision-making and operational efficiency. The advent of data products has brought with it the need for expert management. This means having clearly defined roles and strategies to ensure their success. For those developing these products, understanding business objectives and possessing domain knowledge are becoming as crucial as technical prowess.
Beyond the management of data products, the data science field itself is undergoing a transformation. The role of a data scientist is diversifying and expanding. Take for example the rise of ‘citizen data scientists’ \— technically savvy individuals without formal data science training who can use advanced analytics tools. This democratisation of data capabilities is highlighting the importance of collaboration and interdisciplinary teams in extracting value from data.
Specialized roles such as data engineers and machine learning (ML) engineers are becoming more prominent. Data engineers focus on the infrastructure and architecture that makes data collection, storage, and analysis possible, while ML engineers specialize in creating the algorithms and models that predict future trends. Each plays a distinct yet complementary role within the broader data science context.
As the field evolves, the need for professional development and continual learning is essential. To remain competitive and effective, organizations and individuals must adapt and upskill to meet the demands of these new multidisciplinary teams and ever-evolving technologies. It’s an environment that attributes success not just to individual expertise but also to the ability to work cohesively within diverse teams.
Frontiers of Innovation: New Paths in Data Science
The dynamic landscape of data science never ceases to evolve, continually pushing the boundaries of what’s possible. Graph-based analytics has become a formidable tool in exploring intricate networks, offering unparalleled insights into connections that traditional analytics might miss. This sophisticated approach is proving invaluable for fields requiring nuanced understanding of relational dynamics, such as fraud detection and social network analysis.
As we pivot towards distributed systems, the data mesh architecture is emerging as a transformative model. It decentralizes data management, advocating for a paradigm shift in data accessibility, collaboration, and ownership. By empowering individual teams, organizations are experiencing a notable surge in agility and innovation within their data ecosystems.
The advent of continuous learning models is perhaps one of the most significant strides in AI. These models are designed to learn and adapt on-the-fly, ensuring their precision doesn’t falter as circumstances change. It’s a leap towards AI systems that are not just reactive but truly responsive to the ebb and flow of data streams.
Advancements in natural language processing are bridging the gap between human complexity and machine understanding, allowing for more intuitive interactions between us and our digital counterparts. Simultaneously, federated learning is championing privacy by learning from decentralized datasets without compromising sensitive information — an ethical stride in collaborative AI.
Blockchain’s role in ensuring data integrity is gaining recognition. With its capacity to forge verifiable audit trails, blockchain technology is fortifying trust in data science practices, anchoring data integrity and ethical standards.
In conclusion, these developments encapsulate the enormous strides taken toward enhancing efficiency, understanding, privacy, and trust in data science. Each innovation marks a step along a new and exciting path — one that holds the promise of transforming the way organizations and industries leverage data. As we venture forward, embracing these advancements will not only spur further innovation but also assure that the use of data remains ethical, secure, and incredibly impactful.