Artificial Intelligence, or AI, often feels like a concept pulled straight out of a futuristic sci-fi movie. In simple terms, AI refers to machines that are designed to mimic human intelligence. This doesn’t mean a robot that looks and acts exactly like a human, but rather systems that can perform tasks which typically require human thought processes—like learning, reasoning, problem-solving, and even understanding natural language. In this blog let’s find out what are the key differences between AI And Machine Learning
AI’s roots stretch back to the mid-20th century with key pioneers like Alan Turing, who is often considered the father of computer science and AI. The famous Turing Test, developed in 1950, was an attempt to define whether a machine could exhibit intelligent behavior that’s indistinguishable from a human. This laid the groundwork for future developments in the field.
At its core, AI encompasses a wide range of concepts and techniques. It includes machine learning, natural language processing, robotics, expert systems, and neural networks. Each of these areas works together to create systems that can perform complex tasks autonomously.
The applications of AI are vast and diverse. Think about your daily life—virtual assistants like Siri or Alexa, recommendation engines on platforms like Netflix and Amazon, autonomous vehicles, and advanced diagnostic tools in healthcare. AI is everywhere, quietly making our lives easier, more efficient, and often more fun.
Looking ahead, the future of AI is both exciting and a bit daunting. Experts predict major advancements in areas like personalized medicine, smart cities, and even AI-driven creative arts. However, with these advancements come important ethical considerations and questions about the future role of AI in society. Balancing innovation with responsibility will be key to harnessing the true potential of AI.
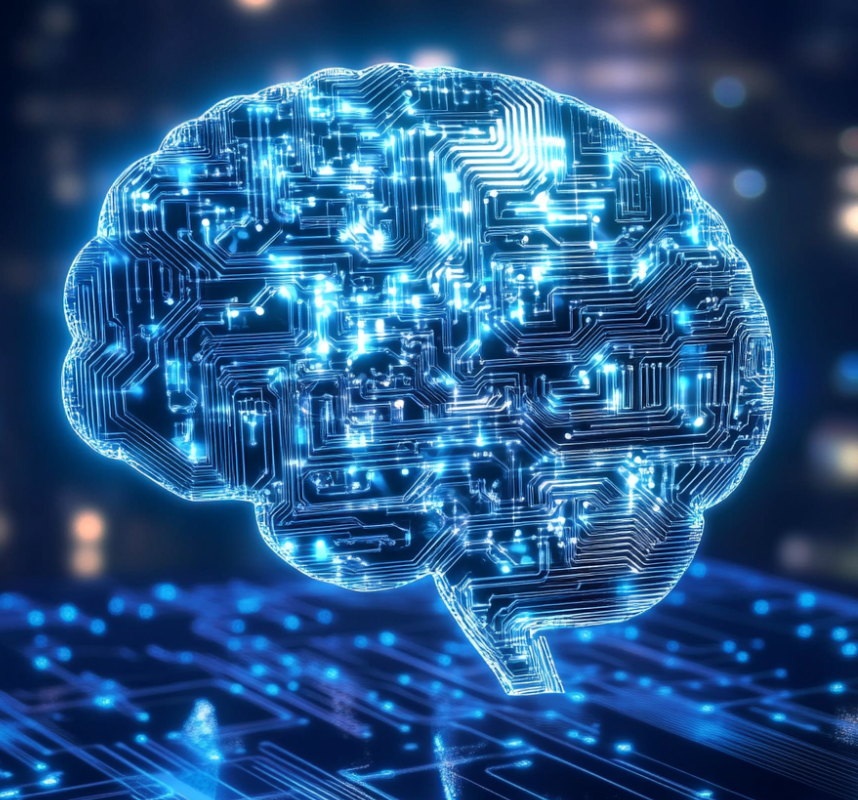
Machine Learning Unveiled
Machine Learning (ML) is often the backbone of AI, focusing on building systems that can learn from and make decisions based on data. Unlike traditional programming, where specific instructions are coded into the computer, ML enables systems to learn patterns and make predictions by analyzing data. So, you’re providing the machine with information, and it figures out the rest—pretty neat, right?
To get a grip on ML, you need to understand its three main types: Supervised, Unsupervised, and Reinforcement Learning. Supervised Learning is like teaching a child with flashcards; you give the algorithm labeled examples until it can generalize to new, unseen data. Unsupervised Learning, however, involves finding hidden patterns in data without predefined labels, like discovering clusters of similar items. Reinforcement Learning is akin to training a pet, where the algorithm learns optimal actions through trial and error, receiving rewards for desired behaviors and penalties for undesired ones.
Different algorithms power Machine Learning, each with unique strengths and applications. Decision Trees, Neural Networks, Support Vector Machines, and K-Means Clustering are just a few examples. Each algorithm serves specific purposes, whether it’s classifying emails, recognizing speech, or predicting stock prices. Understanding which algorithm to apply to which problem is part of what makes Machine Learning so fascinating and impactful.
ML’s applications are broad, cutting across various industries. It powers recommendation systems on streaming services, improves fraud detection in banking, personalizes marketing strategies, and even contributes to medical diagnostics. By learning from past data, these systems can predict future trends and behaviors with remarkable accuracy, driving efficiency and innovation.
Despite its incredible potential, Machine Learning does come with challenges. Data quality is crucial; poor or biased data can skew results and lead to ethical issues. Additionally, ML models often require extensive computational power, and their ‘black box’ nature can make it hard to understand how decisions are made. Overcoming these challenges involves ongoing research, better data practices, and more transparent models.
AI vs. Machine Learning: Core Differences
AI and Machine Learning are like siblings—they share common traits but are distinct in many ways. Understanding their differences is crucial for anyone diving into this fascinating field.
At a fundamental level, AI is the broader concept. Imagine AI as the entire universe of intelligent technologies, encompassing various subfields, including Machine Learning. Its goal is to create systems that can perform tasks usually needing human intelligence, whether that’s understanding language, recognizing patterns, making decisions, or solving problems.
Machine Learning, meanwhile, is a subset of AI. Think of it as one planet in the AI universe, focusing specifically on systems that learn from data. While AI can involve rule-based systems crafted by human experts, ML relies on algorithms that improve autonomously through exposure to data. So, every ML system is an AI system, but not every AI system uses ML.
When it comes to techniques and methodologies, AI includes expert systems, logic programming, and probabilistic reasoning. ML zeroes in on pattern recognition and statistical models. These methodologies often require heavy computational resources. Traditional AI might involve pre-programmed rules, while ML leans on data to ‘train’ algorithms, fostering adaptability and precision.
Scope and goals differ significantly as well. AI aims for overall intelligence and versatility, creating systems that mimic a range of human cognitive functions. Machine Learning focuses on optimizing specific tasks and improving performance over time. You could say AI aims to replicate broader human-like abilities, whereas ML strives for mastery in targeted areas.
Real-world examples help illustrate these differences. AI applications include everything from chatbots like customer service bots to autonomous vehicles. On the other hand, ML shines in areas like email filtering, recommendation engines, and predictive analytics. Both technologies often work together to create powerful, integrated systems, but understanding their unique strengths can help you better leverage their capabilities.
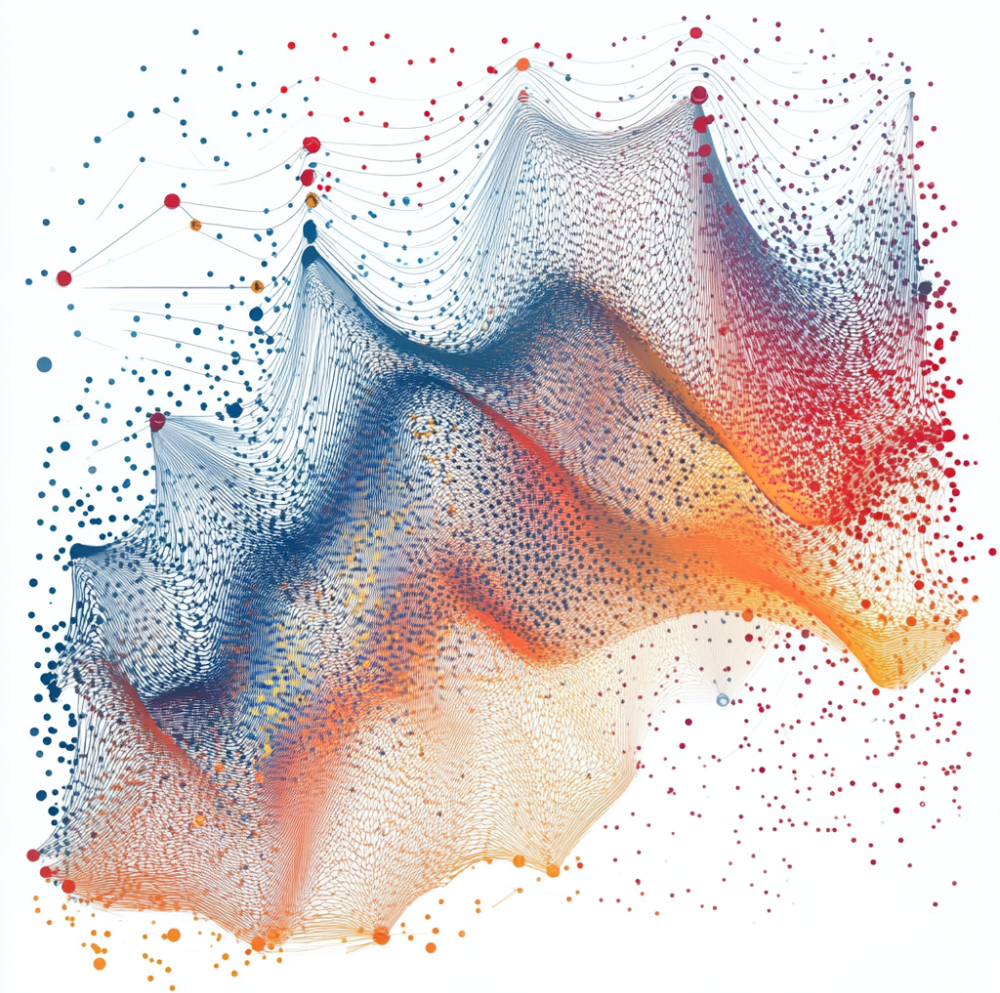
Impact on Industries and Everyday Life
AI and Machine Learning have permeated almost every aspect of modern life and industries, transforming how we work, live, and entertain ourselves.
In healthcare, AI-powered diagnostic tools and personalized treatment plans are revolutionizing patient care. Machine learning algorithms can analyze medical images, predict patient outcomes, and identify potential health risks with remarkable accuracy.
The financial sector is benefiting hugely from these technologies. AI-driven fraud detection systems and automated trading platforms are enhancing security and efficiency. Machine learning helps in creating personalized banking experiences, assessing credit risk, and even preventing financial crimes.
Retail is another sector seeing a massive shift. Recommendation engines tailor shopping experiences to individual customers, increasing satisfaction and sales. Inventory management systems powered by AI and Machine Learning predict demand trends, optimizing stock levels and reducing waste.
Education is also evolving. Intelligent tutoring systems offer personalized learning experiences, while predictive analytics help institutions identify students at risk of falling behind, allowing for timely interventions.
The economic impact is staggering. While AI and Machine Learning are creating new job opportunities, especially in tech-driven industries, they are also automating many traditional roles, leading to a shift in job market dynamics. The need for new skills is more prominent than ever.
With the widespread adoption of these technologies, there are both benefits and risks. Increased efficiency, personalized services, and innovative solutions are undeniable advantages. However, concerns about job displacement, data privacy, and ethical use of AI and Machine Learning loom large.
Ethical considerations are paramount. Ensuring equitable access to AI technologies, preventing bias in algorithms, and maintaining transparency in AI decision-making are critical issues that need addressing. These considerations will shape the responsible development and deployment of AI and Machine Learning in the future.
Future Trends and Developments
Emerging trends in AI and Machine Learning are pushing the boundaries of what’s possible. One of the major trends is the integration of AI with the Internet of Things (IoT). Smart homes, autonomous vehicles, and connected devices are becoming more intelligent, creating seamless, efficient ecosystems.
Natural Language Processing (NLP) advancements are making human-computer interactions more intuitive. Voice assistants are becoming more context-aware, and translation services are improving in accuracy, breaking down language barriers globally.
Edge computing is another exciting development. Instead of relying solely on cloud computing, data processing is happening closer to where data is generated. This is crucial for real-time applications like self-driving cars and industrial automation, where latency can be a game-changer.
Interdisciplinary advancements are also on the rise. AI and Machine Learning are being combined with fields like genomics, environmental science, and even the arts. This collaboration is driving innovative solutions to complex problems—from fighting climate change to creating new forms of digital art.
Experts predict that the future will see even more personalized and adaptive AI systems. These systems will not just react to user inputs but anticipate needs and offer proactive solutions.
Policy and regulatory considerations are coming to the forefront as well. Governments and organizations worldwide are beginning to establish frameworks to ensure AI technologies are developed and used responsibly. Issues like data privacy, ethical AI, and equitable access are being actively debated and legislated.
While the future of AI and Machine Learning is incredibly promising, it’s essential to balance innovation with responsibility. By staying informed and considering the broader impact of these technologies, we can harness their full potential for the greater good.